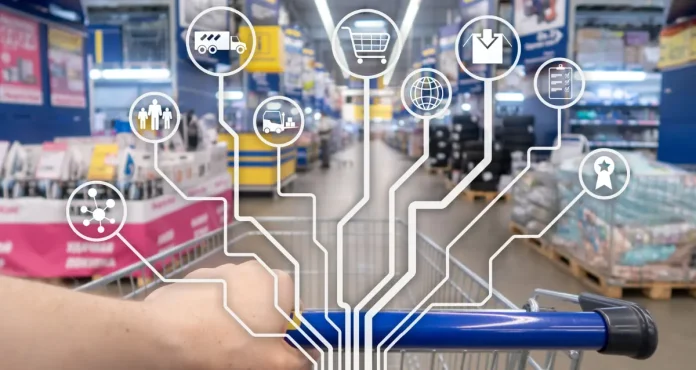
With artificial intelligence and large language models gradually taking over business operations, it’s important to ensure these technologies are up to date. Take into account Forbes’ statistics which reveal that the size of the AI-enabled e-commerce market is projected to be worth $16.8 billion by 2030.
That’s why retrieval augmented generation was introduced. With this framework, LLMs are capable of producing more accurate and relevant content instead of “hallucinating” or making up facts on the spot.
Of course, keeping up to date is also vital for thriving in the e-commerce landscape. In this article, we’ll explore just how significant RAG is in the sector.
What is Retrieval Augmented Generation?
Retrieval augmented generation is a framework developed as an extension to LLMs. The key purpose behind its inception was to overcome the concept of “hallucinations” that were usually associated with LLMs – e.g., models generating data or facts that don’t exist or are incorrect.
A post on retrieval augmented generation at MongoDB gives a clear explanation of how RAG works. The framework allows an AI model to retrieve relevant information from external sources or documents and helps integrate that knowledge into the generated output. Hence, the final information produced is well-informed and factual.
Think of it like a secretary to an executive. Prior to the meeting, the secretary digs through all the relevant documents to present the most useful and pertinent information to the executive. The executive, with the secretary’s valuable input, makes an educated decision based on factual information rather than relying on memory or guesswork.
In the same context, RAG is to an LLM what a secretary is to an executive – assisting in leveraging concrete data instead of “hallucinations”.
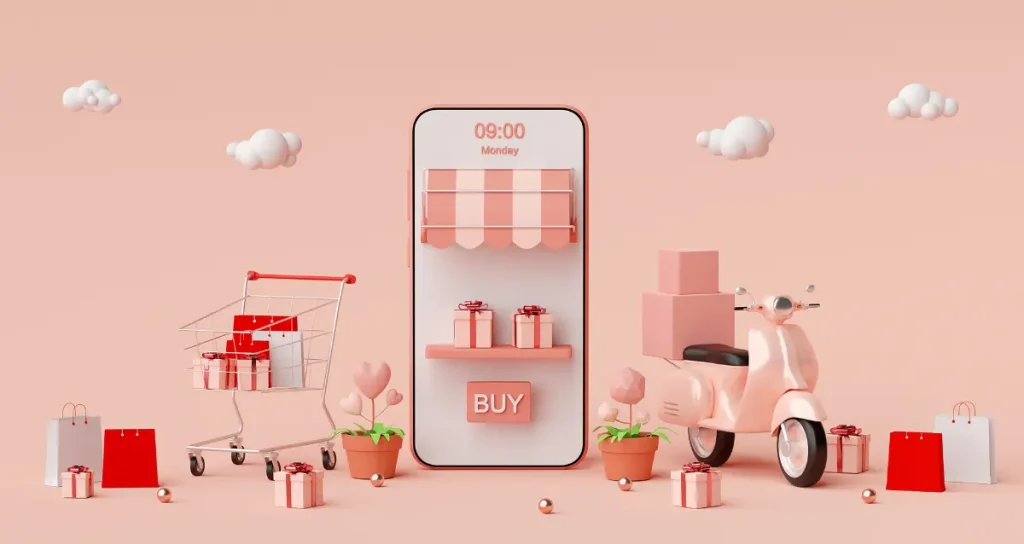
Improving Product Recommendations
One of the most notable impacts of RAG on retail e-commerce is its potential to enhance product recommendations. Generally, recommendation engines depend on information about a customer’s past interactions and preferences.
However, using the RAG approach, these engines can now retrieve comprehensive public data relating to a specific product category, top-rated products, and customer reviews. This allows them to produce more personalized and useful recommendations.
For instance, if a customer has shown interest in sustainable fashion, using RAG, the recommendation engine could pull information from a variety of eco-friendly blogs, sustainable fashion websites, and customer reviews. It could then use this data to suggest products that are not only in line with the customer’s interests but also highly rated and popular within the sustainable fashion community.
Enhancing Customer Service
It goes without saying that it is incredibly important it is to treat customers who you’d like to be treated. RAG is becoming a pivotal tool in enhancing customer service. E-commerce businesses are using RAG-based chatbots or virtual assistants for delivering quick, accurate, and efficient customer service. A great example of this is Shopify’s Sidekick.
According to TechCrunch’s report on Sidekick, the chatbot functions similarly to ChatGPT but is tailored to e-commerce merchants. It can give answers to why a store has low sales or how you can modify store designs.
Traditionally, chatbots have been prone to providing inaccurate information or misinterpreting the context of customer questions. However, RAG-enabled chatbots can pull data from vast resources, allowing a better understanding of customer queries and providing more precise responses.
Streamlining Content Generation
Content is key in e-commerce, hence the need for high-quality, accurate product descriptions. Manually writing these descriptions can be a taxing process.
RAG provides an impressive advantage here; it leverages historical data about similar products to produce accurate item descriptions without the typical time investment. For example, a retailer offering a new line of sneakers can utilize RAG to create descriptions based on past popular sneaker models, customer reviews, and details from the manufacturer.
Content is vital for every level of the sales funnel. RAG can help with this as well, by providing updated information e-commerce businesses can use to persuade leads.
The Bottom Line
RAG is still a fairly new function, and e-commerce companies are racing to leverage its capabilities in their digital operations. Essentially, retrieval augmented generation’s ability to rely on factual and relevant data rather than chances or guesswork holds the potential to revolutionize the way e-commerce operates.
By harnessing the power of RAG, e-commerce businesses can deliver a more personalized, efficient, and trustworthy shopping experience that directly meets the expectations and needs of the modern consumer.
If you liked this article, then we strongly recommend you head over to our Tech section on Covert Voice for more information on the latest tech and gadgets.
FURTHER READING